Artificial intelligence and data analytics impact our lives on virtually every level. The educational context is no exception. Thus arises what we call learning analyticsa research discipline that tries to apply data analysis to improve the teaching and learning process.
Among many other types of studies, there are several research initiatives that work with increasingly sophisticated predictive algorithms that seek to anticipate factors such as the risk of a student dropping out or even the grade that he or she could obtain.
Program to predict
The first works focused on grade prediction were based on the application of a series of pre-established rules on a relatively simple set of facts. More recently, however, the works proposed analyze the entire record of a student’s interaction with her educational platform and use complex neural networks to achieve that grade prediction. In fact, really amazing results are obtained.
For example, in the work presented by Alonso-Misol et al. the performance of different algorithms is compared, obtaining an accuracy of 96% when predicting the grade of an exam. That means that, in 96 out of 100 students, the human puts a grade very close to the one that the algorithm said he was going to put.
It is a relatively recent discipline. It is expected that the results will be better and better, so at some point the following question could be asked: since we have a system that predicts the grade that a student will obtain in the exam with a reasonable margin of error, can we use the prediction as a final grade, and we forget about the exam?
a utopian wish
It sounds tempting, exams are the most hated activity by students. Nor do they enjoy great sympathy among teachers since grading is a really expensive task. Surely, both groups would applaud the end of the exams. However, the reality is quite different and it is very likely that the prediction, now successful, will only produce meaningless numbers if the test disappears.
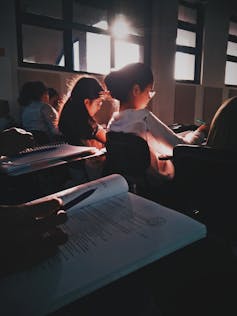
Yustinus Tjiuwanda / Unsplash
This is so because of the way predictive systems that rely on supervised learning techniques work. In essence, the principle of operation is as follows: data from the current course is analyzed and compared with data from past courses. If in past courses there is a pattern of activity that can be related to the achievement of a certain grade, then students who have that pattern in the current course will be predicted to have that grade. In other words, it is very likely that a student will obtain a similar grade to that obtained by students who had a similar interaction with the platform.
Thus, predictive systems will be successful to the extent that the course being analyzed performs equivalently to previous editions of the same course.
To understand this properly, let’s imagine a month-long course in which students have to submit an activity on Friday of each week. There will be students who only generate activity on Friday, to make the delivery. There will be other students who will generate activity throughout the week, with more intensity on Friday. What seems to be clear is that it will be a pattern of weekly activity.
Let us now imagine that a new teacher enters and decides that the activities corresponding to the four weeks are all due at the end of the month. Surely, the activity pattern of the students will change and there will even be students who do not enter the course until the last week. This change in the teaching methodology will make the comparison between the current course and previous courses no longer make sense. As a result, predictive systems will lose their full potential.
Something similar would happen when deleting the exam. Although there are students who have a strong intrinsic motivation to continue with their learning, the extrinsic motivation that an exam imposes is the main motivating factor to continue with the activity in the course. In other words: without an exam, the students would work less in the course and with a very different pattern of work. Predictive systems, therefore, would lose their value.
Other evaluation possibilities
If we want (we would like) to eliminate the exam, then we must think about the whole catalog of alternative activities described in this other article. In any case, whether we call it “exam”, “rubric”, “portfolio”, or in any other way, the activity of the students is highly modulated by the due dates of the activities that count for the grade.
The objective of predictive systems for the qualification of students is, mainly, to detect students at risk of dropping out in order to offer them adequate support. They are also useful for anticipating the resources that will be needed. Among the objectives of predictive scoring systems, however, is not the elimination of the final exam.